Libratus Poker Robot Honored with AI Award
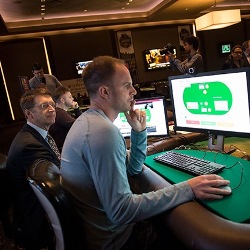
The sophisticated poker robot called Libratus, which dominated both competitions held against human professional poker players this year, recently received the HPCWire Readers’ Choice Awards at the Supercomputing Conference (SC17) in Denver, Colorado. In case you were wondering, HPCWire is the self-professed leading publication for all the latest news and information concerning high performance computing. And yes, you would be right in assuming that most of the content is far above what most of the general public would feel comfortable, or even interested in reading.
HPCWire Readers’ Choice Award
Libratus was developed by Tuomas Sandholm, a Carnegie Mellon professor of computer science, together with PhD student Noam Brown, with its software running on the “Bridges” supercomputer based at the Pittsburgh Computer Center. The formidable program subsequently won the Readers’ Choice Award in the category for “Best Use of AI”, after its winning performance in the Brains vs AI poker competition.
Commenting on the award, Tom Tabor, the CEO of Tabor Communications, the company which publishes HPCwire, said that receiving either a Readers’ or Editors’ Choice Award was a significant honor. Elaborating further, Tabor stated:
“This success signifies support and recognition from the HPC community along with the industries it serves. It is both an honor and privilege to once again engage with our readership and allow their voices to be heard. We extend a sincere thank you to our readers for their nominations and votes and a heartfelt congratulations to this year’s winners.”
Contest Requirements
Libratus took on a quartet of top poker pros in the guise of Daniel McAulay, Dong King, Jimmy Chou, and Jason Les, with the 20 days competition taking place in January at the Pittsburgh’s Rivers Casino. During the No-Limit Hold’em heads-up games, a total of 120,000 hands were played, with the human competitors subsequently losing badly.
Included amongst the techniques used to eliminate luck as much as possible from the experiment was the resetting of chip stacks after each hand to remove the advantages associated with welding a big stack. Also, if someone went all-in and was called before the river, no more cards would be dealt and chips would subsequently be distributed based upon the equity each player had in the hand at the time in order to avoid so-called “suckouts”.
Furthermore, each of the hands was repeated for both human and AI opponent, with the hands reversed so that neither side would receive more lucky deals than the other. For example, if a human player was dealt 8-9 in one hand, and Libratus was dealt K-K, then in another hand Libratus would be dealt 8-9, with the human opponent then getting the pocket kings hand.
Results
The game was played with each competitor receiving a starting stacks of 20,000 chips, and the cash game played with blinds set at $50/100. At the end of almost three weeks of play, Libratus ultimately walked away with $1,766,250 of play money, equivalent to winning $14.72 per hand. Moreover, no human player was able to defeat the AI during this time, with Dong King coming the closest after losing $85,649, an amount much less than his other teammates.
Having crunched the numbers, Tuomas Sandholm then concluded that there was a 97.7% degree of certainty that Libratus had been playing the game more skillfully than his professional human opponents.
Programming
What’s truly remarkable, however, is that Libratus hadn’t even been programmed to make particular moves based upon specific situations, but was instead given several options for each different scenario. By way of example, when holding specific cards and facing certain sized bets, Libratus’s programming would tell it to double the bet 40% of the time, raise by three times 20% of the time, and call in 25% and fold in 15% of these situations. When the competition ended, the computer then went through each of its hand and adjusted its strategy accordingly.
Libratus was trained by Sandholm and Brown by getting it to play billions of hands of poker against itself, and analyzing and adjusting its decision-making algorithm based upon the outcome. Explaining further, Sandhol explained that Libratus wasn’t exactly taught how to play. The algorithms weren’t specific to poker, but rather took “as input the rules of the game and output strategy.”
Brown and Sandholm also made Libratus concentrate on its own strategy and decision making process, rather than trying to identify and exploit weakness in its opponents’ play, as this approach was deemed safer. That is because exploiting your opponent leaves you open to being exploited yourself.
Round 2
Libratus squared off against its human opponents again in April, with one member of the six strong “Team Dragon” being 2016 WSOP bracelet winner Tue Duand. The competition took place in Hainan, China, with once more the AI program scoring a convincing victory. The match was played over 36,000 hands, but this time around there were real stakes involved, with Libratus making a $290,000 profit all told, equivalent to 220 “milli-big blinds” per game.